Hello! 👋
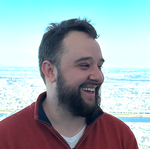
I'm a software engineer who loves building things, learning as I go and sharing my knowledge with others.
Some recent things I've written about:
- I scanned every package on PyPi and found 57 live AWS keys
- Infosys leaked FullAdminAccess AWS keys on PyPi for over a year
- Visualizing how S3 deletes 1 billion objects with Athena and Rust
- CVE-2022-0329 and the problems with automated vulnerability management
And some interesting things I've built: